Deep Dive
The New Frontier in Finance
Explore the transformation of the CFO role and finance organizations and learn how AI and unified platform solutions are shaping the finance toolset, empowering finance teams to transition from data consumers to data drivers.
About the Authors
Principal @ Contrary. Megan invests in companies at Series A and beyond with a focus on fintech, marketplaces, and data. She previously worked at DoorDash in Finance & Strategy and Goldman Sachs in Technology, Media, and Telecommunications (TMT) investment banking.
CEO @ Vareto. Previously, Kat was Head of Product at Ironclad and led product, data science, and finance teams at Meta. She is also a GP at Darkmode Ventures, where she invests in early-stage companies with a focus on developer platforms, AI/ML, and data.
Actionable Summary
- The evolving CFO role – from scorekeeper to proactive strategist. Over the past two decades, the finance organization has evolved from a reporting function to a forward-looking strategic function. The looming recession in 2023 is increasing the pressure on finance teams to play offense, as business units look to finance teams to help them determine the right levers to pull in order to maximize profit, reduce operational costs, and maintain growth. Straightforward transactional responsibilities are now table stakes, with finance workflows moving from one-directional “streams” to iterative “cycles.”
- The evolving finance org – from data consumers to data owners/drivers. As the CFO’s charter evolves from simply managing the business to running the business, so too must their organization. Executives are increasingly looking to their CFOs to develop the right data strategy to help them make better decisions, with many companies investing in solidifying their data analytics and architecture at much earlier stages. Furthermore, there is a shift towards all analytics and operations teams working together in a more integrated way to effectively orient the company on long-term strategic goals and break down information silos between teams. To enable these shifts, CFOs are expanding the scope of their finance teams and restructuring them accordingly in 2023. Some CFOs, primarily in small to mid-market (50-500 employees), now report overseeing data & analytics and revenue operations (RevOps) teams (traditionally under the CTO and CRO, respectively).
- The evolving finance toolset – from spreadsheets to platforms. With recent advancements in AI, a growing shortage of finance talent, and an expanding scope of strategic responsibilities, CFOs are taking a hard look at their tool stack. The finance ecosystem has become inundated with best-of-breed point solutions over the last 20 years. Despite having data integrations, most solutions still could not manage the complexity finance teams needed as the CFO scope continues to grow. A rebundling movement is occurring across categories like expense management, procurement, and HR as ~80% of CFOs expressed interest in switching to a single vendor solution with wider breadth versus multiple-point solutions. In the next few years, we see the most immediate applications of AI in finance further deepening and accelerating the automation that has existed for some time, as opposed to creating something totally different. However, it’s important to recognize that many AI applications will require quite a bit of fine-tuning before they can be safely and effectively used by finance teams.
- How can finance leaders best position themselves for this new frontier? To influence GTM decisions and effectively leverage AI to unlock productivity, finance leaders must invest in (1) aligning finance and business partner workflows and (2) centralizing their data as early as possible. In fact, studies have shown that companies that prioritize data-driven insights are 23x more likely to attract new customers and 19x more likely to be profitable. Beyond planning, finance leaders must demonstrate their ability to be critical partners during execution by guiding CROs and CMOs with real-time data and insights post-planning and pre-close. It’s critical to ensure all of your business teams and your AI-enabled tools are operating according to the same definitions.
- How can founders building in finance best position themselves? To sell to CFOs, founders building in finance must (1) demonstrate ROI for adjacent non-finance departments and (2) build robust data architecture with clear inputs, outputs, and connections to other tools used by finance teams. While enterprises may hold distribution power and data moats, startups have the upper hand in its speed and agility to reimagine workflows. With the recent advancements in API-backed integrations and AI, we believe an exciting new chapter of finance software has arrived. A large opportunity exists for vendors who can deeply embed themselves within finance and business workflows to deliver time savings, break down data silos, and ultimately empower finance and business leaders to operate better together.
Why Now? The CFO’s New Mandate
Never let a crisis go to waste – and CFOs know that better than anyone else. It’s an opportunity to reinvent, reduce inefficiencies, and make better investments to arrive on the other side stronger than your competitors.
Over the last decade, the process of modernizing finance systems has been slowly moving up the list of executive priorities. However, in the last three years, the pressure to play offense has mounted. Now, more than ever, CFOs are laser focused on maximizing profit and improving operational efficiency. There are three key tailwinds at the center of this transformation in the finance org.
1
Shortage of Talent
Across finance and accounting, companies are struggling to retain talent. Accountants and auditors in the US have left the field in droves, causing a 17% decline in these roles between 2020 and 2022, often due to retirement or burnout. In corporate finance, the quality of the talent pipeline has also taken a major hit. Finance teams traditionally relied on former investment bankers or Big 4 professionals. But as these institutions move down the "prestige stack" during university recruiting, companies find that they’re losing strong talent directly to private equity, hedge funds, or startups much earlier in the conversion cycle.
With an expanding scope of responsibilities and increasingly limited headcount as a result of macroeconomic headwinds, CFOs in 2023 are feeling the pain of relying on legacy systems, which solve only a fraction of their evolving needs. Historically, finance teams would hire more analysts to do routine monthly and quarterly processes, but now they are actively exploring tools to automate these tasks and drive efficiency. In short, CFOs need better software to maximize the impact of their short staffed teams.
2
Financial Org Expansion
Some CFOs, primarily in small to mid-market companies (50-500 employees), now report overseeing data & analytics and RevOps teams (traditionally under the CTO and CRO, respectively). The focus of this expansion is to:
- Break down data silos between teams. Given the loosely defined structure at startups, data & analytics and RevOps teams naturally lend themselves to finance activities than those under the CTO and CRO. With nascent processes, information flow across individuals and teams is free-flowing. As the business matures, functions get bigger and inevitably grow farther apart.
- Reduce workflow gaps. Historically, finance was seen as an output, not as an input to GTM performance. As the finance function takes on a more strategic role, finance partners increasingly find themselves working hand in hand with RevOps teams over projects like deal desk and commissions. Having multiple teams separated by systems and processes gets in the way of each function scaling together.
The expanded scope increases pressure to invest in tools that (1) integrate well with the adjacent departments’ tool stack, and (2) enable finance/accounting orgs to scale their responsibilities while automating traditionally administrative tasks (e.g. reporting, consolidation).
3
Rise in AI Applications
86% of financial services executives plan to increase AI-related investments through 2025 to drive productivity and operational efficiency. CFOs are focused on ensuring the finance department has the right tech stack to provide leverage for their expanding responsibilities. In Q4 2022, Deloitte’s CFO survey indicated two key priorities for finance executives over the course of 2023: (1) 84% of CFOs agreed that they will use automation to free people up for higher-value activities, and (2) 79% intend to embed more automation into operations.
We will use automation/digital technologies to free people to use their talents for higher value activities in 2023
We will embed more automation/digital technologies into operations in 2023
Strongly disagree
Somewhat disagree
Neither agree nor disagree
Somewhat agree
Strongly agree
Source: Deloitte Q4 2022, North American CFO Signals survey
In the past, many companies have been slow to adopt novel finance tools as they had either (1) already made significant investments (e.g. time, money, personnel resources) into legacy solutions, or (2) the productivity gains did not outweigh the migration risk. In an interview with Contrary Research, a Head of Finance at a $10+ billion consumer social company stated:
“As the saying goes, ‘nobody gets fired for buying IBM.’ But it’s different now. We don’t have the resources to throw more heads [at managing these systems]. I’ve evaluated and passed on a lot of new generation finance tools, but I think AI could be the ‘unlock’ this time. Whoever can show me a quantifiable ROI across not just finance, but data and ops will be a winner.”
The need for agility in the face of volatility has forced many CFOs to rethink the role of the corporate finance function. CFOs are now looking beyond accounting close to providing more dynamic support to the organization. The ability to pivot quickly, guide strategic decisions for business partners, and reduce cycle times is increasingly vital.
From Streams to Cycles
Traditionally, the CFO had three main tasks: financial reporting, investor relations, and compliance. CFOs were viewed as number-crunchers who operated behind the scenes. They told you “no” when there wasn’t enough budget and reported financial results after the fact. Strategic decision-making was left to the rest of the C-Suite. In other words, the CFO was confined to a “sign-off” role.
The Changing Role of The CFO
From technical and fiduciary expertise with a narrow financial focus...
... To a business partner with strategic and operational focus, leading a low cost finance organization
Minimum Requirements
- Management reporting
- Controllership and compliance
- Investor relations
- Using financial data to challenge the business and contribute to operational decision making
- A catalyst for driving business transformation
- Link strategic objectives with the financial goal of the business
Source: HighRadius
As such, the finance workflow has been one-directional and reactive. Finance’s job was to monitor how a company’s money was being spent and ensure the company didn’t run out of money, while marketing and sales were seen as the revenue generating teams. In the old order, finance was just a collections function.
Today’s CFO responsibilities look more like that of a “Chief Performance Officer.” The new persona for CFOs is no longer that of an accountant focused on reporting, but rather an operational leader focused on understanding. The directive has shifted from managing the business (by executing routine monthly and quarterly financial processes) to running the business (through day-to-day analysis and operational decision-making). Straightforward transactional responsibilities are now table stakes.
Most value
Most time and resources spent
1: Strategic Finance
e.g. product planning and pricing, resource allocation, capital structure M&A
2: Financial Planning
e.g. budgeting, forecast
3: Reporting and Compliance
e.g. monthly close, audits
4: Record Keeping
e.g. reconcilliation, recording transactions
5: Transacting
e.g. sending payments, checks
Source: a16z
Most value
Most time and resources spent
Strategic Finance
e.g. product planning and pricing, resource allocation, capital structure M&A
Financial Planning
e.g. budgeting, forecast
Reporting and Compliance
e.g. monthly close, audits
Record Keeping
e.g. reconcilliation, recording transactions
Transacting
e.g. sending payments, checks
Source: a16z
In an interview with Contrary Research, a CFO at an HR software company described his evolving role this way:
“My role looks more like a COO these days. I spend way less time with accounting and more with operators developing our go-to-market strategy. I’m dealing with other department heads all the time, and I need a tool stack that enables enterprise-grade collaboration functionalities and talks to the other tools that sales and RevOps are using.”
As such, modern finance workflows are moving from one-directional “streams” to iterative “cycles.” Finance was once seen primarily as a planning to reporting function. Now, finance is brought in to bring alignment between cross-functional teams while guiding business partners with real-time data and insights post-planning and pre-close. Companies are transitioning to more agile approaches like Beyond Budgeting, Rolling Forecasts, and Zero-Based Budgeting. These approaches may seem like no-brainers, but in fact, 45% of companies still report relying on only traditional budgeting (i.e. static annual plans).
Source: Contrary Research
To address the expanded scope of responsibilities, some CFOs – primarily in small to mid-market (50-500 employees) – now report overseeing data & analytics and RevOps teams to bridge organization alignment. More generally, there is a shift towards all analytics and operations teams working together in a more integrated way to effectively orient the company on long-term strategic goals and break down information silos between teams.
Data & Analytics
Poor data quality costs the US economy up to $3.1 trillion annually. Dysfunctional financial reporting was estimated to cost US businesses $7.8 billion in 2022. Despite the proliferation in data tools, many companies are struggling to still utilize data to run the business.
This remains a massive problem. In fact, over 70% of enterprises are still behind in their ability to create value from data. 87% of data projects never even make it to production. Data projects that do not involve consumers of data, like business teams, in the design phase often result in lower adoption. Including data teams as partners or integral parts of the business team can encourage better alignment and faster decision making.
RevOps
Businesses are selling into more channels and business models than ever before, making revenue management a significant challenge. As this complexity grows, so does the need for a cohesive department to accelerate growth and provide line of sight into revenue. In a 2022 survey, 95% of C-Suite executives in high-tech and software said they have implemented RevOps-related initiatives or are in the process of or considering doing so. By housing the RevOps function with GTM finance partners, CFOs can ensure that both departments are anchored on the same post-sales goals to drive change across sales, marketing, and customer success.
That said, organizational alignment doesn’t solve all problems. The tools used in the respective departments today may not support a unified workflow.
In an interview with Contrary Research, a strategic finance lead at a $10 billion+ HR software company described the connection between finance and RevOps this way:
“There are huge workflow gaps with existing finance and RevOps tools right now. For example, in CPQ, CRM, and billing systems (i.e. RevOps tools), if a customer comes online, they could be 6 months out from starting the tool and ARR doesn't go live until then. How do you set the onboarding period and make sure it’s booked correctly through [your financial systems]? We had to build internal tools to figure this out.”
In addition to operationally balancing financial and revenue reporting, there are limits to integration between these systems. Sterling Snow, the SVP of Revenue at Divvy and now Bill.com, made this clear:
“Every time you launch a product, there's a lot of manual work between finance and revenue teams to clean up and map the data, including ARR, closed/won, and billings. It takes a lot of time to align on core definitions.”
The increased emphasis on fully-integrated tools in the finance org represents the beginning of a new chapter in finance.
The Evolution of Finance Tools
Over the past four decades, the evolution of finance tools has spanned across four acts:
- Legacy On-Prem Systems
- Cloud-Based Point Solutions
- Data Integrations Everywhere
- The Rebundling
To understand the future opportunity for finance tools, it’s important to understand the strengths and weaknesses of incumbent solutions and where they fall short of modern finance needs. Let’s unpack each stage of evolution below.
Evolution of Finance Tools
Pre-1990 to Today
Less data
More data
Pre - 1990
Ledger Paper
Slow processing and expensive storage prevents anything beyond 1-person solutions
1990 - 2000
Spreadsheets
Microsoft Excel becomes the dominant tool, but a siloed approach makes integration near impossible
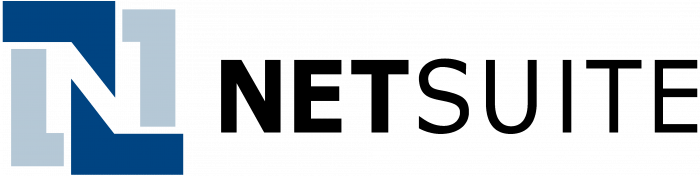
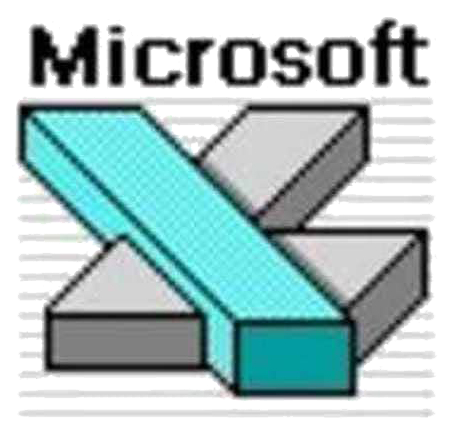
2000 - 2010
Point Solutions
Point solutions disaggregated functions from legacy systems to provide consistency, but becomes less agile at scale
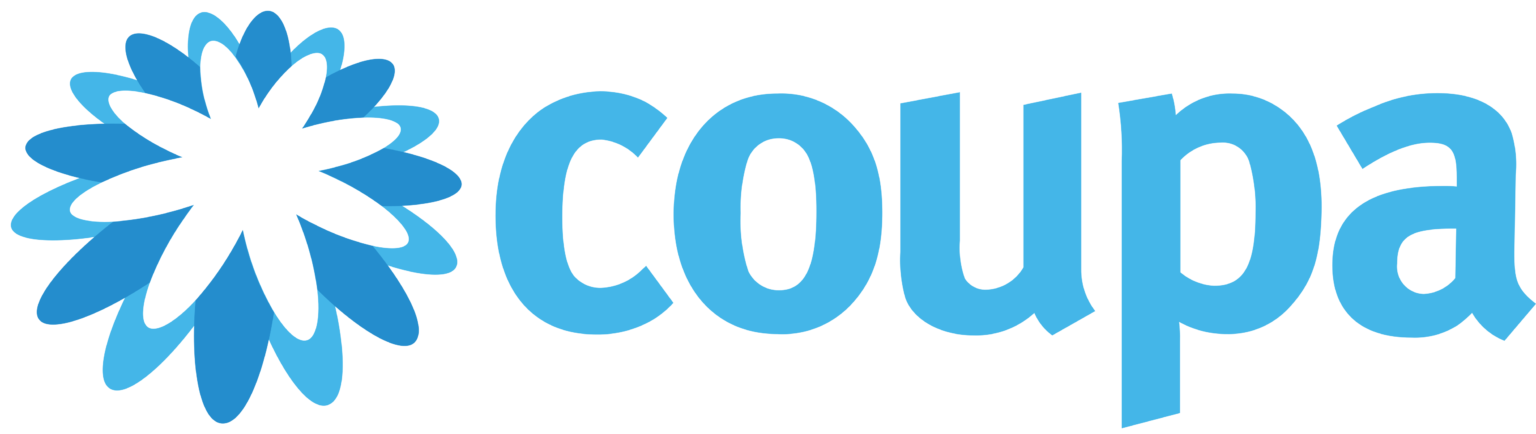

2010 - 2020
Cloud & SaaS
Native SaaS apps take advantage of data integrations to connect to point solutions
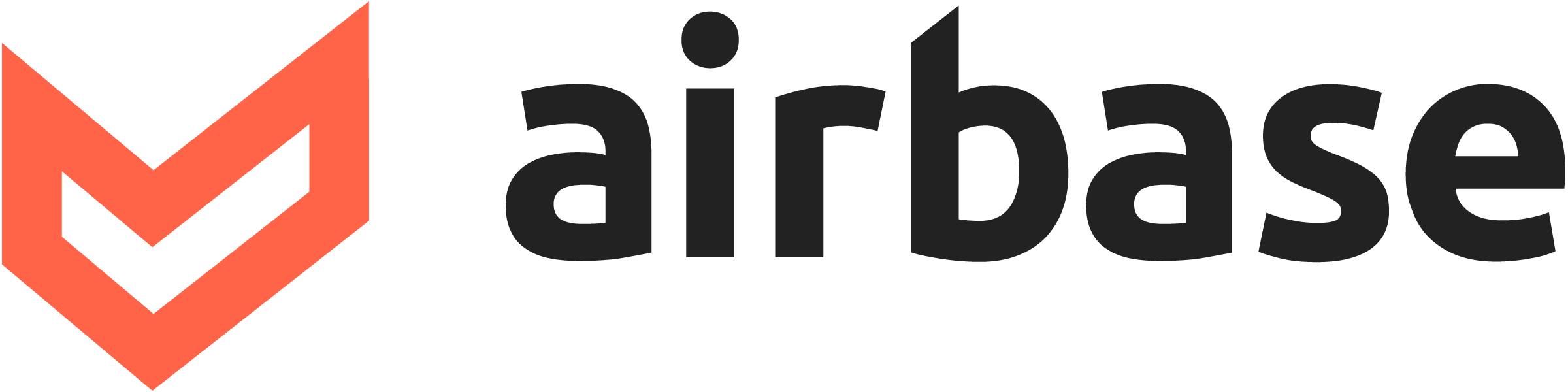
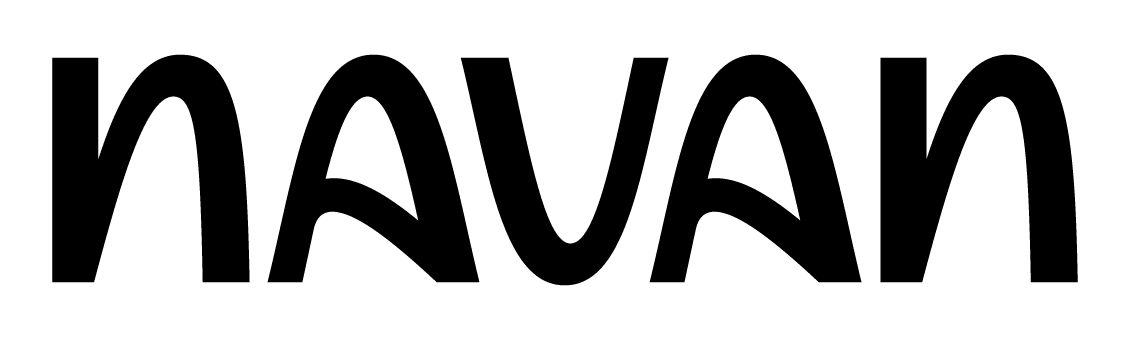
2020 -
Rebundling
Explosion of data leads to inconsitency, pushing execs to rebundle their financial apps into one unified platform
Source: Contrary Research
Act I (1980s - 2000)
Legacy On-Prem Systems
Legacy finance software primarily consisted of enterprise resource planning (ERP) and enterprise performance management (EPM) systems. Leading companies in the space included Hyperion (Oracle), SAP, and IBM TM1. Although ERP systems date back to the 1960s (known as material resource planning), it wasn’t until 1990 that Gartner officially coined the term “ERP.” ERPs fully integrate the entire business across one single database in order to create agile adaptations to the demands of the market for the schedules of MRP and MRP II systems.
These products were on-premise solutions that typically took more than 6 months to implement and involved large hardware costs, with total cost of ownership sometimes exceeding $1 million in the first year. This significant upfront investment, combined with slow processing and expensive storage costs, made such solutions accessible to large enterprises only. The widespread use of Microsoft Excel also limited meaningful integration across different tools as local workflows were siloed.
Act II (2000 - 2010)
Cloud-Based Point Solutions
ERP systems continued to evolve throughout the 2000s. First offered by NetSuite in 1998, the cloud ERP gained widespread adoption in the mid-late 2000s. Businesses could now access critical data from any device with an internet connection. Cloud solutions meant companies no longer needed to purchase and maintain hardware, reducing the need for IT staffers and leading to easier implementations.
Along with this shift to the cloud, second-generation tools disaggregated functions from legacy systems to create an ecosystem of best-of-breed point solutions with tools such as Anaplan, Workday, Coupa, and Expensify. Take expense management for example. While some ERPs do have expense management features, they were seen as an afterthought to the core solution. As a result, user experience was suboptimal. A standalone solution that integrated with native ERPs allowed greater flexibility and insight.
But the strength of point solutions also proved their greatest weakness: they performed only one function. As a company scales, a weak infrastructure made up of Excel spreadsheets and a few point solutions starts to break down. Finance teams are left wrestling with multiple platforms, user permissions, and disparate data sets. This is where the real problems arose.
Act III (2010-2020)
Data Integrations Everywhere
Between 2010 and 2020, the world saw a rapid increase in data volumes. The amount of data created, captured, copied, and consumed globally increased roughly 50x from 1.2 trillion GB to 59 trillion GB. The rise of cloud data warehouses, like Snowflake and Databricks, and data transformation companies, like dbt Labs and Fivetran, enabled companies to store data at scale and increase the volume of accessible data. With the cambrian explosion in data combined with accelerating cloud deployment, CFOs were now faced with new questions, including:asas
- How should the business capture data (structured and unstructured) across the finance value chain?
- What new tools can scale with the business while integrating well with the existing tech stack?
- How does the finance team partner with business stakeholders to drive insights and make decisions?
To address this, third-generation tools such as Bill.com, Navan, and Floqast focused on native data integrations, analytics, and automation capabilities to alleviate tedious tasks, allowing finance teams to focus on strategic activities. These solutions were significantly less expensive than second-generation solutions, appealing to mid-market buyers. However, adoption of data tools often outpaced that of finance tools, while finance’s scope continued to grow.
Although most point solutions advertise integrations to eliminate data silos across tools, marketing is often different from reality. Every veteran finance leader has a horror story of some major integration gone wrong, resulting in the finance tool they purchased becoming shelfware.
In an interview with Contrary Research, Albert Wong, the Director of RevOps at Alloy, pointed out how disparate financial systems still are:
“Every financial tool says they have integrations with financial systems, but it doesn’t fully fix the issue. The tools are still disparate. We still had to build Salesforce connectors to pull into Google Sheets, and even then, some custom fields from Salesforce don’t travel across the system. This results in more manual reconciliations and delays accounting close.”
These issues led to the genesis of API companies such as Merge, Rutter*, and Finch. Even so, the best-of-breed finance tools still build their own native integrations to key systems such as Netsuite to reduce dependency risk and support the full array of complexity that finance teams require.
Act IV (2020 –)
The Rebundling
With the rise in function-specific point solutions and the explosion of data over the last decade, the finance tech stack has become highly fragmented. Despite the multitude of offerings, many finance leaders expressed interest in consolidating their stack. In one survey, ~80% of CFOs expressed interest in switching to a single vendor that provided an integrated offering versus multiple best-of-breed point solutions. However, incumbent vendors still own a significant market share. Within EPM, Oracle, SAP, IBM, and Anaplan together hold 56% market share. In travel and expense (T&E), SAP holds 52% market share, followed by Expensify at 7%. Fourth-generation vendors seek to address a new type of customer persona consisting of agile teams that are digitally native and looking to make faster decisions.
Compared to the previous generations, fourth-generation finance tools focus on user-centric design, low cost, shorter implementation times, and self-service features. Many of these companies have focused on selling to SMBs (i.e. companies that do not have an established finance tech stack in place yet) with the goal of scaling with their customers as they grow. Another strategy, taken by upmarket competitors, is to sell a “wedge” solution into mid-market and enterprise companies, and later expand to provide a broader set of services and displace legacy incumbents.
Fourth-generation finance companies have also used data as the wedge for expansion across adjacent categories. We’re already seeing a rebundling movement happen across categories, in line with bundling sentiment above. Ramp* moved from a corporate card (taking on American Express) to expense management (Concur), bill pay (Bill.com), working capital financing, and SaaS buying. Zip started from intake-to-procure and expanded into payment. Tropic started in procurement then moved into SaaS management and contract management. Rippling started in HR and IT management before releasing a suite of finance products, including corporate cards and expense management.
While legacy solutions like SAP Concur focused on building deeper enterprise functionalities within a vertical, newer tools focused on owning the “transaction layer” for the CFO department, rapidly deploying new horizontal products.
As Tomasz Tunguz puts it:
“A simple way of dividing the software world is system of record vs. workflow application. Systems of record are the single source of truth about a particular department or company. The next shift in SaaS will see startups leverage their workflow roots into disrupting systems of record by changing the buying process. The data they will aggregate from this usage will provide unique insight that preceding systems of record cannot furnish.”
The turf wars are just beginning.
Building Blocks of the Finance Stack
In line with the rebundling movement and the shift from “streams” to “cycles,” newer generations of tools focus on (1) bridging financial and operational data to become the system of record and (2) providing deeper collaborative functionalities for both finance and business partners. Below, we have highlighted rising players making a bid to serve modern finance needs across four core workflows.

Source: Contrary Research
Systems
Enterprise Resource Planning (ERP)
The ERP is the system-of-record which contains the accounting general ledger and offers non-financial resource planning capabilities (e.g. supply chain, project management, inventory). Smaller businesses tend to opt for Quickbooks (Intuit) and Xero. Larger companies graduate to enterprise-grade solutions like NetSuite (Oracle) or SAP. Some aspects of the traditional ERP have been effectively unbundled into offerings like Coupa or Bill.com. Coupa is a business spend management tool that competes with SAP Ariba or Oracle’s NetSuite SCM module. Bill.com has carved off aspects of a traditional ERP that would be served by products like SAP Concur or Oracle’s Financial Module.
Data Warehouse
Traditionally, management viewed data warehouses as cost centers under the CTO. While many companies do have internal processes to store and collect data, the sheer quantity of data produced in the last decade has forced CFOs to turn to external sources. By centralizing the data warehouse and using it as the source of truth, finance and non-technical teams can more quickly query and analyze multiple data sources. Access to historical data increases performance transparency and agility for decision making. Popular data warehouses include Snowflake, Amazon Redshift, and Google BigQuery.
In an interview with Contrary Research, James Lee, the VP of Finance at Productboard, outlined the complexity in setting up a fully functional data management system:
“The hardest thing is ensuring data quality and metric standardization. Companies often wait too long to set up a business intelligence/analytics department or data lakes. Having a good setup allows you to democratize knowledge and drive decision speed. Everyone needs to stare at the same numbers.”
Business Intelligence (BI) & Analytics
While the volume of data has grown exponentially, the ability to understand that data and derive insights from it is still a complex process. Finance has stepped in to wrestle data into a usable format, both for financial and operational analysis. Some data analytics stacks can be as simple as a Tableau or Looker dashboard built on top of a data warehouse, like Snowflake or Redshift. As finance sought to become more strategically informed, other tools have emerged to provide specific financial analysis, such as Digits and Subscript.
Planning & Forecasting
Financial Planning and Analysis (FP&A)
FP&A tools enable finance teams to plan, forecast, report on, and analyze their business. First-generation companies like Anaplan, Adaptive, and Planful primarily served as repositories for financial plans, but did not eliminate friction in the cross-functional work that goes into the act of planning, reporting, and decision-making. As such, finance teams still wrestle with a combination of CSVs, Excel/Google Sheets, and ad hoc SQL outputs. The idea of automating and streamlining these things is a more modern need which only newer tools are trying to solve.
Although Microsoft Excel is still the most popular FP&A tool, newer platforms are focused on consolidating user workflows into one place by creating access controls, collaboration functionalities, data integrations, and web-based modeling and reporting. These tools take one of two approaches: (1) augment spreadsheets (OnPlan, Datarails, Cube), or (2) replace spreadsheets entirely (Vareto, Pigment, Mosaic). The former approach will be more familiar to Excel-heavy users, but can be limited in features and functionalities as these companies do not have total platform control.
Workforce Planning
Labor can constitute ~70% of total operating expenses, yet headcount planning is notoriously difficult given the multiple stakeholders, complex data sources, and competing priorities involved. Newer tools aim to be the source of truth that syncs across employee data systems, including human resources information systems (HRIS) and applicant tracking systems (ATS). Unlike spreadsheets, these tools allow companies to plan their teams, track hiring, forecast attrition and budgets with role-based access controls. Notable players engaging the workforce include ChartHop, Knoetic*, and TeamOhana.
Benchmarking
The pandemic accelerated equity shifts in the workplace and dislodged historical spending trends. Pave* and Compete provide more transparency on employee compensation. Growth and marketing KPI benchmarking companies like Varos help finance teams compare metrics against similar companies to decide which KPIs to double down versus spend time fixing.
Execution
Accounts Receivable (AR) & Revenue Management
Managing revenue has become an increasingly complex exercise for companies as they start to balance a variety of different models for monetization. Managing the entire revenue cycle, and in particular the process of accounts receivable (e.g. money you’re owed), has become an exercise in complexity. For traditional subscription businesses, companies like Zuora, Chargebee, and Recurly are typical platforms for managing AR. In addition, the rise of usage-based pricing models has given rise to companies like Metronome and Orb.
Accounts Payable (AP) & Spend Management
Managing a company’s expense account can lead to death by a thousand cuts for many businesses. The average company spends $343K a year just on SaaS subscriptions. Legacy players like Avidxchange and Bill.com helped businesses pay faster, but newer AP and spend management companies are focused on helping businesses pay less. They do this by contextualizing drivers for vendor and employee spend, automating accounting workflows, and streamlining reporting and bill pay. Notable players include Ramp*, Brex, Vic.ai, and Glean.ai.
FinOps
According to a 2022 survey, ~50% of businesses are struggling to control cloud costs, partly due to lack of visibility into usage. Reducing cloud costs has become a top priority with 81% of IT leaders directed to reduce or take on no additional cloud spending. “FinOps” is a cross-functional practice that brings together finance and engineering to optimize cloud spending, gain more financial control and predictability, and deliver products faster. Although this category has existed for more than a decade with companies like Cloudability, CloudHealth, and Apptio, increasing complexity in cloud infrastructure led to new players like Vantage, CloudZero, and Zesty.
Procurement & SaaS Management
Today, the average company uses over 130 SaaS solutions, with SaaS spending now the third largest cost item behind payroll and real estate. This, combined with the decentralization of purchasing decisions, has created immense friction for modern businesses. Tracking, managing, and negotiating software spend remains a clunky, complex process.
Procurement identifies cost savings and negotiates on the company’s behalf. SaaS management helps organizations organize, optimize, and orchestrate its SaaS suppliers. The categories have converged over the past two years as players look to complete the SaaS buying lifecycle, from discovery to vendor management. Notable players include Productiv, Zylo, Tropic, and Vendr.
Payment Operations & Cash Flow Management
Payment operations address the lifecycle of money movement, including transaction initiation, approvals processing, payment failure resolution, and transaction reconciliation to bank statements. Historically, large back office teams managed these time-intensive and manual processes. With the proliferation in payment methods (e.g. buy-now-pay-later (BNPL), real-time payments (RTP)), cross-border transactions, and new business models (e.g. usage-based, geo-based, volume-based), payment reconciliation is becoming a massively complex process. Companies like Modern Treasury, Proper, and Nilus are tackling transaction automation at scale.
Cash flow management tools integrate with a company’s bank accounts and financial tools to track cash inflows and outflows in real-time, and provide visibility into the business’s cash position. Notable companies include Agicap and Trovata. The two categories have also converged recently as players look to build a unified platform to orchestrate and manage money movement.
Record Keeping
Bookkeeping & Tax
Most companies have an accounting close and tax preparation process. If all goes well, the close is a routine process that does not attract much attention from management. But it’s a completely different story if the numbers are late—or wrong. Speeding up the closing process (typically 5-10 days) enables executives to make faster decisions and frees finance teams to focus on more important strategic priorities.
However, these processes are extremely intensive, as accounting and tax teams must work with finance to reconcile transactions and file tax forms under tight deadlines. Historically, companies like Blackline and Avalara have been the legacy tools of choice for close management and tax management, respectively. Companies like Floqast, Trullion, Puzzle, and Anrok have emerged to take advantage of the way these processes are changing.
Payroll & Benefits
Payroll and benefits platforms simplify the management of a global workforce, from recruiting and onboarding to compensation and compliance. Broader HR platforms like Rippling and Gusto aim to be the single system of record across departments. As the pandemic fueled the distributed workforce, companies like Deel, Remote, and Papaya Global helped businesses hire both independent contractors and full-time employees and streamline payroll. Although these systems are primarily owned by HR, finance teams often involved as payroll expenses constitute a significant portion of spend.
Compensation Management
Sales compensation software supports the creation and implementation of sales incentive plans, captures sales data, and calculates and tracks commissions. Legacy solutions could calculate monthly commissions easily, but intra-month, sales and GTM teams often found themselves tracking deals in Excel and then making manual adjustments to determine sales payouts to quota-bearing reps. These issues were exacerbated as companies scaled in size and payout structures got more complicated. Newer commissions tools facilitate collaboration between finance, ops, and HR and integrate with the data warehouses that contain compensation inputs. Legacy tools like Xactly or Anaplan have been used to manage this process in the past, but popular tools including CaptivateIQ and Spiff have emerged over the last few years.
Financing
Banking & Treasury
Neobanks primarily serve startups and offer traditional banking services (e.g. checking/savings accounts, financing, treasury) coupled with modern technology, such as APIs that allow customers to build customizable automations into their workflows. After Silicon Valley Bank’s collapse, companies like Mercury and Brex experienced billions of dollars of deposit inflows.
Big tech companies have their own dedicated corporate treasury departments which engage in critical capital return programs to drive shareholder return. Apple, for example, has its own asset management company called Braeburn Capital to handle its cash. However, this function does not exist at the SMB level today. Emerging players focused on standalone treasury product include Vesto*, Arc, and Meow.
Cap Table Management
Cap table management platforms help companies manage their equity cap tables, debt facilities, 409a valuations, employee stock compensation and more. Companies in this space include Carta or Pulley for equity management, Finley for debt management, and Standard Metrics for portfolio management.
AI Applications in Finance
CFOs have been urged for years to modernize how their teams work. With recent advancements in AI, coupled with a growing shortage of finance talent, CFOs are taking a hard look at their tool stack, and AI could be the differentiator this time. 86% of financial services executives plan to increase AI-related investments through 2025 to drive productivity and operational efficiency.
For example, Marie Myers, the CFO of HP, stated:
“The bottom line is that the numbers are substantial. [AI is] probably the most agile moment in productivity that I’ve personally seen in my career aside from the smartphone and the internet. … This is a really significant management of change. Finance professionals like rigidity and they like structure. This disrupts everything. I do believe you need a different level of skill and agility in your organization, and even potentially a different sort of structure, to enable productivity gains at speed.”
Where is the Power in AI?
Some of the biggest impact that AI will have on finance will be within platforms. Leveraging AI is unique from other platform shifts in that, unlike the shifts to mobile or cloud, most companies aren’t starting from scratch. Instead, the ability to build a model typically requires a significant amount of specific training data. The more users a model has, the more effective it becomes. As such, platforms with large existing user bases benefit tremendously.
As a result, some of the first movers you see in AI for finance are incumbents. Established platforms, like Workday and Microsoft, built their own in-house AI solutions. Bloomberg’s launch of BloombergGPT is a LLM built specifically around financial data, taking similar advantage of the company’s existing data to build the model with 50 billion parameters.
The Co-CEO of Workday, Carl Eschenbach, emphasized the company’s efforts to more deeply integrate AI:
“We’ve built AI and ML deeply into the platform. What most companies are doing, because they haven’t built it into the platform from day one, they’re bolting it on. If you want to bolt on AI, you have to go pay for that.”
While enterprises can hold distribution power and data moats, startups have the upper hand in its speed and agility to reimagine workflows. Brex partnered with OpenAI and Scale AI to launch Brex Empower, which provides insights comparing spend across similar companies and industries or per-employee efficiency. Ramp* Intelligence, powered by GPT-4, leverages its existing corpus of transaction data for vendor price intelligence, accounting copilot, and contract extraction and negotiation. Things are developing quickly. But beyond AI chatbots, what could change?
What Does AI Change?
In the next few years, we see the most immediate applications of AI in finance further deepening and accelerating the automation that has existed for some time, as opposed to creating something totally different. The various levels of automation drive change through three phases: (1) delivering time savings through automation of transactional tasks, (2) breaking down data silos across disparate workflows and tools, and (3) ultimately empowering finance and business leaders to operate better together.
On one end, technologies like robotic process automation (RPA) automated over 42% of finance activities – typically basic transactional tasks. In fact, activities such as general accounting operations, cash disbursement, and revenue management topped 75% in its ability for automation. On the other end, AI goes one level higher by enabling humans to make better, faster decisions.
AI applications can be spread across a spectrum of productivity gains, from individual to organizational. Decision support (e.g. chatbots) is often the first step. However, increases in individual or departmental productivity are table stakes. Finance leaders are optimizing for organizational productivity. For example, for forecasting, AI could go beyond dry variance analysis to look for past correlation with holiday period, macroeconomic situations, current events, and provide a starting point that makes sense for finance, data or ops teams to get involved. Companies with the stickiest applications generally start building for a champion department, then build down the spectrum to enable productivity gains for adjacent business partners.
Build AI into Finance Cycles
Indivisual Productivity
Organizational productivity
Decision Support
AI Application
Insight Generation
Impact
Knowledge democratization
Notable Announcements

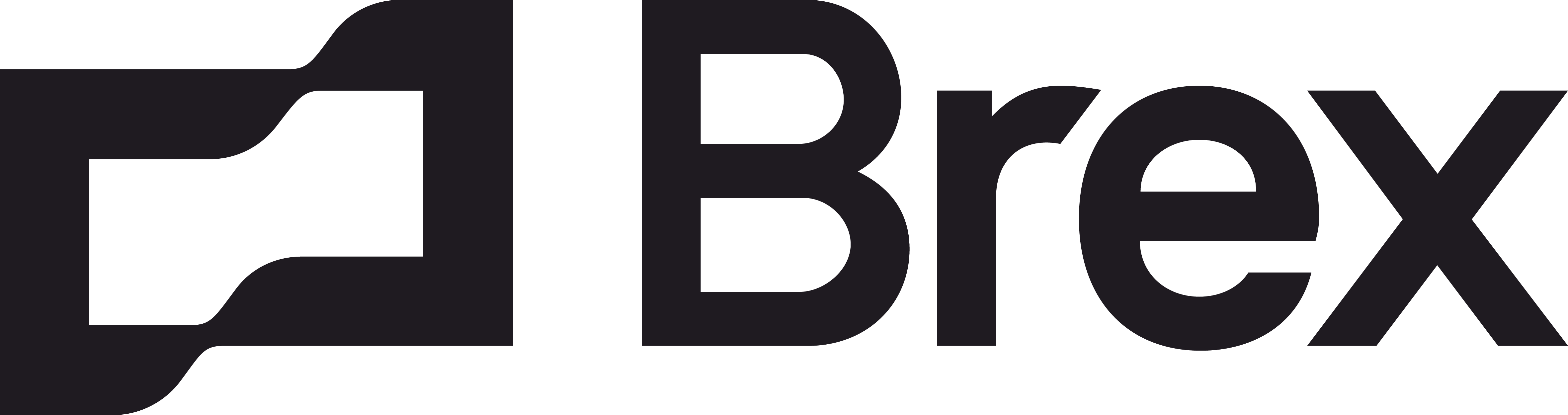
Forecasting
AI Application
Modeling Copilots
Impact
Faster, more efficient modeling experience
Notable Announcements
Billings & Collections
AI Application
Predictive Analytics
Impact
Reduced revenue leakage
Notable Announcements
Spend management
AI Application
Unstructured data extraction
Impact
Spend less. Pay faster.
Notable Announcements
Fraud detection
AI Application
Data enrichment & classifications
Impact
Reduce fraud and credit losses proactively
Notable Announcements

Contract management
AI Application
Data matching
Impact
Accelerate contract negotiation and mitigate risk
Notable Announcements



Monthly close
AI Application
Anomaly detection
Impact
Reduced manual reconcilliations
Notable Announcements

Source: Contrary Research
Here are some initial applications we're seeing built into the finance cycle:
- Decision support: Leverage AI to automatically answer questions about data, provide narrative summaries, and suggest adjacent questions or analyses.
- Forecasting: Modeling copilots can help finance teams build models faster and with fewer errors, similar to how Github Copilot helps engineers write code more efficiently. In addition, AI can help compare multiple scenarios and understand the metrics impact from drivers to enable faster iteration during planning and forecasting cycles.
- Billings & collections: Collections can be reactive, but automation can enhance the collections process by forecasting which customers will pay invoices and when, among other flags for at-risk accounts. ML can be leveraged to predict different outcomes.
- Spend management: AI can analyze the extracted data from documents (e.g. invoices, receipts, or contracts) to identify patterns and trends, enabling finance teams to gain insights into spending patterns and identify areas where efficiency can be improved.
- Fraud detection: Businesses lose roughly 5% of their revenue every year due to fraud. ML algorithms can be trained with historical data to suggest risk rules, which can block or allow certain actions, such as fraudulent transactions or identity theft.
- Contract management: AI can help organizations read contracts under negotiation highlighting areas for review, discover areas of concern for compliance, and provide recommendations for points of negotiation.
- Monthly close: AI-powered tools could help with reconciliation by detecting anomalies across transactions and payment systems and flagging discrepancies across systems.
What are the Risks of AI?
It’s important to recognize that most AI applications for finance today are exciting dreams, but largely still just dreams today. Finance is a highly regulated industry. As such, this side of the world has been relatively slow to adopt generative AI due to concerns around data leakage, security, and privacy. A recent Salesforce survey of 500+ senior IT leaders found that 71% believed enterprise use of generative AI could expose company data to new security risks.
Here are a few risks to be aware of while using AI for finance applications:
- Incorrect information: There are multiple causes including poor or outdated training data, user error (e.g. not entering AI prompts correctly), or even AI hallucinations. This can result in ethical or legal consequences. For instance, if a conversational AI advises a CFO with false information, the CFO could make poor decisions with severe downstream consequences (e.g. massive financial losses, market instability).
- Sensitive data mishandling: Without proper data governance, AI may not have the necessary security and privacy measures to handle sensitive customer information, such as personal identifying information (PII), transaction details, or financial account data. If mishandled, this can result in identity theft, financial fraud, and material reputational damage.
- Lack of explainability: While AI/ML can help with forecast accuracy and other use cases, there is often a black box problem when it comes to explaining these results. In a driver-based model, you can clearly point to the inputs, outputs, and calculations. In an AL/ML model, there might be thousands or even millions of variables and no neat formula to connect the dots. This can cause issues for decision-making, management oversight, and compliance, especially in highly regulated industries.
Finance leaders must develop a roadmap for AI implementation and establish governance, security, access, and controls to its data.
- Who should have permission access to underlying data?
- What tasks/questions should or should not be delegated to AI?
- When should you rely on AI?
- How do you determine an appropriate confidence level for an AI answer?
- How do you measure success and quantify ROI of an AI implementation?
While AI capabilities are likely to be transformational, the question of what aspects of finance they will transform is still an open question. AI can help alleviate tedious tasks, but it does not yet fully understand business context, know the right questions to ask, or how to present cohesive answers.
Braving the New Frontier
In the past decade of economic “good times,” it was easy to put together back-of-the-envelope hiring plans and throw more heads at manual reporting processes where needed. Now the tides have turned. With the macro environment worsening, a growing shortage of finance talent, and an expanding scope of strategic responsibilities, CFOs are taking a hard look at their tools. Even cash-rich businesses are looking to uplevel their tech stack to unlock automation, enhance decision-making, and improve the bottom line.
Looking ahead, how should finance leaders and companies building finance tools best position themselves for this new frontier?
What should finance leaders keep in mind?
- Align finance and business partner workflows: The finance function is no longer seen as just an output, but rather an input to GTM performance. In fact, studies have shown that companies that prioritize data-driven insights are 23x more likely to attract new customers and 19x more likely to be profitable. If your finance org is still primarily a collections function, how do you earn a seat at the GTM table? Build trust with your CRO and CMO as early as possible. Beyond planning, demonstrate that finance teams can be critical partners during execution by guiding operators with real-time data and insights post-planning and pre-close. As the business matures, functions get bigger and inevitably grow farther apart. It’s important that finance teams remain the connective tissue across the organization. Even if finance leaders do not have direct oversight over data & analytics and RevOps teams, leaders should constantly look for ways to reduce workflow gaps by bridging the respective systems and processes.
- Centralized and structured data is a prerequisite: As the saying goes, “garbage in, garbage out.” Imagine a CFO asking an AI chatbot to pull revenue data without having first defined what “revenue” means, or sales teams trying to model customer segmentation scenarios without shared data logic for account classification. Finance teams must implement data governance as early as possible. Ensure all of your business teams and your AI-backed tools are operating according to the same definitions when it comes to dimensions (e.g. business lines, customer segments, departments) and core KPIs (e.g. ARR, LTV). At scale, it’s not only more painful to consolidate, but also more expensive.
What should founders building for finance keep in mind?
- Demonstrate ROI for adjacent departments: As CFOs continue to align finance and business partner workflows, tools must demonstrate tangible ways that help both finance and stakeholders work together more efficiently. Improving finance productivity alone is not enough. Who else are you enabling? How will your tool help cross-functional partners collaborate with finance?
- Start with data, not machine learning: Many startups are quick to tout “AI/ML for finance.” But applying ML effectively requires robust data architecture to support your data flows. What data will you have access to, and what new data will you be creating? For example, will your software be a new system-of-record for a specific use case, or are you focused on consolidating existing datasets and layering on your own insights on top? Be clear on the data structure that underlies your software, and how it will interact with other tools (e.g. via integrations). If your tool’s data seems siloed, finance teams will be reluctant to adopt it. Also, without a clear data foundation, you won’t be able to effectively leverage AI.
Make no mistake: selling to CFOs is no easy task. However, while enterprises may hold distribution power and data moats, startups have the upper hand in its speed and agility to reimagine workflows. With the recent advancements in API-backed integrations and AI, we believe an exciting new chapter of finance software has arrived. A large opportunity persists for vendors who can deeply embed themselves within finance and business workflows to deliver time savings, break down data silos, and ultimately empower finance and business leaders to operate better together.
Thank you to Jeff Epstein, Jeff McCombs, Mitesh Popat, Taylor Otstot, Mika Kasumov, Mark Hawkins, Eddie Lievens, and Ehud Gelbum for their thoughts and feedback on this piece.
*One or more Contrary affiliates have invested in companies in the financial tooling market, including Ramp, Pave, Vesto, and Knoetic, which are included in the market map above.
Download The New Frontier in Finance as an ebook
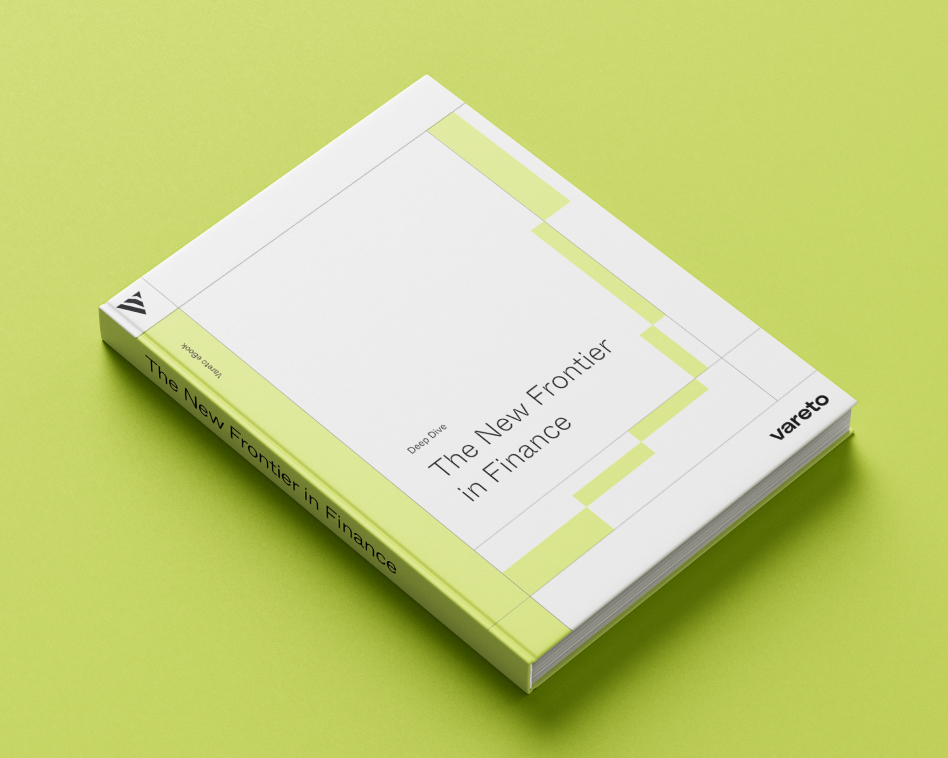